Data²'s Game-Changing AI for Energy & Defense
0:00 And we're back on what the funk I took probably about a month away from podcasting, which was kind of nice. I do have a day job that pays me money. So sometimes I should focus on that instead of
0:12 just putting out podcasts. But I love it. And I'm personally really excited about this one for a few reasons. New client of funk futures, I put out a press release recently about this data squared.
0:23 That's data two dot AI. We've got Matt Kilker and John Bruton, two of the leaders over at the organization data squared. But also you guys are doing AI in oil and gas, obviously, data squared dot
0:40 AI. But AI is a hot topic right now. I don't think anybody really knows what they're doing in the oil and gas basis relates to AI. But you guys have a lot of ideas We've got some military
0:53 backgrounds, some oil and gas backgrounds. working with some government entities, working with oil and gas operators, sports fans, located all over the place, really kind of cutting edge, I
1:06 think, for what's happening in oil and gas, and personally really excited to see where things go with data squared. So this is a little bit different. Typically it's only one person in me. I've
1:15 got a couple dudes on today. So we'll start with you, Matt. And then we'll go to you, John. I'm gonna put you right on the spot. Who is Matt Kilker? That's a whole good question. Inquiring
1:27 minds want to know, right? So thanks for having us, appreciate it. I serve John as the chief revenue officer for DataSquared, focused primarily on the energy sector. We've got a lot of fantastic
1:41 people that John will tell you about. That's on the government side of our business that we're kicking off. But I'm a native to Colorado You're up on the front range? Yeah, that's something we
1:55 haven't really chatted about yet. Where about, where'd you grow up? You grew up in Brighton, the thriving metropolis.
2:03 So, enjoyed our time there, stepped out and went to college in Oklahoma State, got a degree in biosystems and agricultural engineering. Really thought my path was gonna lead me towards design and
2:16 tractors and doing cool stuff in agriculture. And somehow, some way I ended up in oil and gas in the OFS space as a design engineer for Baker Hughes. And just like all of us in oil and gas, we
2:31 took our promotions and travels around the country in and out of Houston and Oklahoma and back and forth to Colorado a few times. And now we're in the DFW area, just outside of Fort Worth, wife and
2:44 three daughters, enjoying them go crazy and trying to keep sanity. After I stepped away from the OFS space about five years ago, I spent the the biggest focus of my time on the water management
2:59 space in the midstream space with a few operators and excited to see how we can make digital revolutions come to life here.
3:12 Nice, so you grew up in Brighton, which is just east of here. I'm in Lafayette, close by. Why'd you decide to go to Oklahoma State? Was it the agriculture school that you wanted to get your
3:22 education in? Or what drove you to the sooner state? Yeah, absolutely. So it was, frankly, a really good engineering school appreciated the program that they had put together. I had no idea
3:37 what agricultural engineering was when I started there, was focused on mechanical. Enjoyed the space that we got there. But if you would have asked me in high school, are you gonna head to
3:48 Oklahoma State? It probably wasn't in the cards and like most. Gentlemen, I followed my bride down there. It was her idea to step down and it was a great place for us to go start our life together.
4:01 Nice. So how did you meet the data square team?
4:06 Rees out to John on a LinkedIn post where we started talking about his business development needs and fit the bill from a project perspective that I think is a niche that we'll probably get into a
4:19 little bit in the water management space and just have had a fantastic time here. It's a great individual and a great team to work with. Oh, that's cool. So for everybody listening, you can get
4:30 jobs on LinkedIn just by reaching out to people directly, probably not by applying, but just reaching out directly. We'll get back to more with you, Matt. Now, Mr. Bruton, who is? John Bruton.
4:44 This is an easy question, a non-complex person. John Bruton, CEO, founder of DataSquared, but I. life began for me in Pasadena, Texas, just south of Houston. Born and raised, used to NATO,
4:60 ghostros, go rockets. I think my journey into the stuff that we're going to talk about today is a bit of a winding path and it started with the military. I was an enlisted airman in the US Air
5:16 Force, served here in the States and also overseas, but I started my career in the military as an honor guardsman, which is a very nice job that doesn't have a lot of utility outside of the
5:35 military, but a very cool job to have while you're in the military. A couple of years then, I was like, man, I need to go back to school. I didn't finish college before I joined the military
5:45 Officers were living great lives, and I thought, man, that looks good. So, I'm going to go back to school. So, So, I. I got out of the military under a palace chase agreement, which
5:54 essentially allows you to get out of active duty, transfer to the reserves while you go back to university. And that's what I did. The intent was for me to go back and to the military as an officer.
6:07 And when I transitioned out of active duty, I transitioned into the petroleum zylos and lubricants job for the US Air Force. Small scale refining operations, liquid nitrogen production, JPA
6:21 management, working with local refineries and different things in the places that you're at. And that really led me to oil and gas, a bit of a winding road to get there. I did have some family
6:34 that was in oil and gas, but for the most part, nobody really had a deep root in the industry or in any companies. Went to a hiring fair with somebody And oddly enough, I went just with my body to
6:49 the hiring fair.
6:52 But my name in that, eight months later, I was an employee at BP, training to be a field engineer and basically a company man on rigs. So that's how I got my start. A couple of years after that,
7:05 I transitioned over to Chevron.
7:08 We had a shared training center, Chevron and BP did, it was the drilling training alliance, which was a great little place in Houston And I would always show up there and all the BP folks were
7:23 looking like they weren't having a great time and all the Chevron folks looked like they were having a really good time. And the demographics of the companies were wildly different. Chevron was
7:34 really, really young at the time. And so it seemed like a really good fit. And so I ended up applying, going over to Chevron. worked in a myriad of capacities there and a number of places started
7:47 in California, transitioned to the global group during my time in the global group. I worked in Scotland, Nigeria, South Africa, Brazil, the UK, but I moved to Thailand, lived in Angola for
7:59 several years, then transitioned back to the States. And it was really at that point, I'd sort of worked in every facet of upstream and sort of midstream by this time, and this is 15 years in.
8:14 And Chevron, the majority of my career, there was basically like a mercenary, I'd show up, try to figure out what was going on, problem solved in places. And most of it was through the
8:23 implementation and use of like new technologies and solutions as a byproduct of that. I spent the last decade of my time at Chevron, really paying attention to what was happening in the market,
8:33 what were the cool tools things coming out and how we could use them to improve our operations, improve our predictability, you know, recover more costs. I'm sorry, reduce our cost and recovery
8:44 more revenue. And so, the byproduct of that, I ended up going to school again at Harvard joint program through the Paulson School of Engineering and the Harvard Business School. Been 18 months
8:57 there, made some good friends, ended up moving to Australia,
9:06 running a portion of
9:13 a company. Over there, there was a advanced analytics, machine learning, AI solutions development firm called Quantium, and after about six months working over there, I thought, you know, I
9:15 don't know why I'm doing this for somebody else, I need to start my own company. And so that's really how we got data squared, but Houston native, Big Big Sports fan, oil and
9:25 gas, lifer for the most part, and really, really focused on how we can use some of the new things that are coming to market to change, how we operate, and really, our efficiency curves, just as
9:39 an industry, I think are gonna be effective in a really positive way as we start to roll down this path. Yeah, I appreciate the answers from both of you guys, and I would just comment on AI in oil
9:51 and gas, which I wanna dive pretty deep into here. I see a lot of AI companies that, of course, wanna get into oil and gas, but might not have the subject matter expertise, which as we all know,
10:02 is a non-starter. And then I think there are oil and gas companies themselves who may not have the internal technological horsepower to implement AI in a way that they want. So talk a little bit
10:13 about the thesis behind data squared. When did you guys start the company? Where are you effectively all based? And what are you guys doing? Like AI is so ubiquitous and generic. Like, let's
10:28 talk a little bit more specific effects. What are the use cases that oil and gas companies apply for artificial intelligence in their business. Cool, well, I'll pick up at least the front end of
10:40 that. We started DataSquared in
10:47 let's say,
10:49 July of '23, that was essentially the first time the founder cohort came together and said, all right, there's a lot of new stuff we've been talking about things. We all met up in Vegas, which
11:00 was great. Set in a room in the mirage and said, all right, let's chart out our future. Are we gonna build a company? Are we gonna be consultants? We're gonna build products, what do solutions
11:09 look like? How are we gonna impact the market? And it really settled on sort of like three key themes. Out of the founder group, we either served in the military, we're oil and gas lifers in some
11:23 capacity or both, or studied together. And so really tight knit group, but we had a couple of key vertical markets that we understood really, really well, and we could apply our subject matter
11:34 expertise to And so we really centered on - All right, let's really focus on using our experience, our understanding of these tools and how they can impact these sectors and try to develop some
11:46 initial product solution facing approach. What our use case is try to define these things. And through that, we essentially got to the point where we were targeting high reliability industries,
11:59 really boils down to like energy, defense, finance, healthcare We don't know much at all about healthcare, so that's sort of a thing that's sitting off to the side at the moment, all there's deep
12:11 applicability in that space. And so we really wanted to focus on energy and defense as our primary approaches and our understandings of these places and some low hanging fruit at the time that we got
12:22 started was abandonment.
12:26 One of the cool things about generative AIs, you can give it sort of two versions of something and you can say, hey, what are the differences? What are the similarities? Which one comes before?
12:35 If there's temporal elements defined in these documents, like start dates and end dates and wares and who's?
12:44 As a byproduct of that, we said, all right, let's see what we can do with abandonment because that's a really complicated problem to solve from an oil and gas engineering perspective. Was an
12:51 abandonment SME and wrote all kinds of standards for Chevron, especially in the Asia South business unit around how we do that work. It's a complicated workflow because you really have to reconcile
13:05 asset histories over a really lengthy period of time in most instances, especially for dealing with mid-continent North American assets that have been around for nearly 100 years. And there was this
13:21 light bulb moment where we fed in a bunch of information and it was. handwritten documents that we had scanned. It was old scan, typed reports, new electronic form documentation, and we started
13:34 to really use this to reconcile not only sort of the asset history, but like what is the current state of this target asset? Like what's in the whole, what problems does it have? Where is it right
13:47 now? And we found pretty quickly that we were able to do that and do that with a high degree of efficacy But this is a really funny thing. It's like, you know,
13:58 a really good outcome, but completely unintended consequence. Because of the problem that we tried to solve, it's really differentiated from both a data perspective and just sort of a historical
14:09 perspective. You have structured data, semi-structured data, unstructured data in the form of documentation and in some instances high frequency data that you can leverage to make an informed
14:19 decision about what that asset is at that time. Because of that, we essentially set up a system where we have to cross-reference every single thing that we see to determine what is the current state
14:30 of something. As a byproduct of that, we sort of got into this testing protocol that we wrote when we were developing our platform that allows us to essentially determine the connections of any
14:42 piece of information on any elements we can define, the who's, the what's, the wins, the where's And as a byproduct of that, we can define essentially what's happened and how it happened. And
14:58 that was a really interesting point because what that all does when you put it together is it creates explainability. And this is something the industry struggles with in an exhaustive capacity right
15:12 now because there are no commercially available platforms and generic of AI tools that produce fully explainable, transparent and trusted results is just a little fact.
15:24 we kind of stumbled on a way to make that happen. And it's hyper repeatable. And it doesn't matter what we pointed out. It doesn't matter what data we use. It doesn't matter what LLMs we use. We
15:37 figured out a way to make that happen. And it's sort of through taking an engineering approach to problem solving that we manufactured this outcome. And it really was like the old pen board with
15:50 yarn, you know, tying all these things together and saying, How do these things work? But in a really smart and efficient way. And that really set us on the path that we're on right now, which
16:01 is high reliability industries focusing on high efficacy rate decision-making, suggestions, interrogation, investigation that creates really, really effective outcomes. So I'm gonna stop there.
16:16 I'll leave Matt, jump in. Feel free to add any context you'd like on your side Dumb it down for us, Matt. Yeah, so John's brilliant gets on tangents that we have to be timed out a second, but
16:29 appreciate all of it. I learned something every time. I think, so I wanna look at a perspective from the user. If I'm acquiring a business, if I'm trying to look
16:59 through a data set that may be disparaged, every oil and gas company out there has records that aren't seamless, that we've stored in flat file systems, we've stored in boxes, we've got Excel
16:59 spreadsheets everywhere. As a good engineer, we should have Excel spreadsheets in every folder of our domain. And so when we start to think through how do we operationalize that data set, it
17:11 becomes overwhelming at times. So the advantage that I see that we're trying to do different is trying to create those connections between not just the data set itself, but the data itself. So each
17:25 individual attribute that we have within that data set is meaningful and it takes countless years and hours of very skilled individuals trying to decipher that and create connections between those
17:39 data sets that can either optimize a system that can produce predictive results going forward, can create plans and scenario models that if we just allow it to sit in that flat file, it becomes very
17:55 cumbersome to try to get through. The tool that we've developed has the ability to decipher all of that and create those connections between data sets and allow us to have tangible results in very
18:09 complex scenarios with disparate data sources. I like that and now talk to me a little bit about how you interact with the data. So obviously there's this component of you've got Excel spreadsheets
18:21 and you have
18:24 you know, public data sets available through state agencies or some of the big, you know, subscriptions that companies have, you have internal data sets, you've got messy data, some that needs
18:33 to be OCR sitting in boxes. Okay, so you work your magic and you go through a process that figures out this data and places it in boxes and allows it to interact together. How then does the human
18:46 interact with that data? Is this through like asking questions of it through a chatbot? What does the workflow look like then on a daily basis for the ops engineer or production lead that wants to
18:59 ask questions of the data? This is a good tandem question 'cause I'll address it from a data model perspective and then Matt can talk about exactly how we interact from a user perspective. From a
19:13 data modeling perspective, we're not using SQL
19:19 databases traditional relational databases.
19:22 because they lack a level of fidelity that you need for these systems to work really, really well. There's a couple of concepts that you'll hear in the market from AI systems, generative AI systems,
19:35 like retrieval augmented generation, which is effectively a way to convert words and proximity to numbers and then correlate these things and then use that to find the shortest path between two
19:46 things, essentially similarity Tools so you can say like these things are grouped together and they're related to one another is a byproduct of that. That can only get you so far and it's where a
19:58 lot of the systems that are commercially available today start to fall down. They start to really perform poorly in a couple of different ways because of the structure of how they work, they're all
20:10 statistical in nature. We don't need to get into the math behind that, but at the end of the day, you're taking guesses that are based on probabilities that are associated to numbers that are
20:20 derived. to proximity and it's this complicated chain of logic that gets applied when you do hit chat GPT or any other large language model and ask a question. But we found out pretty early is that
20:33 if we use knowledge graph data models, we were able to almost pave a road for that reasoning because they're tangible in nature. You can see how one element of the data model is connected to another
20:44 element. You can actually see the strength of that connection. And as a byproduct of that, it's in research now, it's like really prevalent. People are talking about graph, retrieval augmented
20:55 generation because it gives you a step change in effectiveness. You go from what would be effectively a 30 efficacy rate on returns for traditional RAG methods to roughly 60.
21:08 The key differentiator for us is that once we figured this out, we figured this out about a year ago, is we also started just doing testing to figure out how we could make that model more robust.
21:19 And it's kind of through this that we define this workflow where we both sort of test all of this information and then enrich this information in a multitude of ways. And so what our grapharact
21:31 solution looks like that's being sort of very polite to our solution and not giving it the right amount of deferences I think Berrybond's 73 Homer season like all juiced up on growth hormone and
21:48 steroids and like that's what we're building from a data model perspective. Like this thing is exceptionally robust, hyper contextualized, semantically embedded at multiple levels of metadata tags
22:00 that
22:02 allow you to parse out every single element within the data model As a byproduct of that, we can create full transparency and clarity about how information is being leveraged by a model, we can
22:15 ground the model to the fact base that we give it and as a byproduct of that. the efficacy rates of what we deploy from our review platform are exceptionally high. And so that allows you to
22:26 interface with these models in a couple of really unique ways. One is through natural language and then one is through user inspection sort of of the graph database model itself. There's gonna be
22:36 like 07 of people in the world that are gonna look at the graph and be like, I really wanna dig into this. And then everybody else is gonna be like, let's just use words because that makes a lot
22:47 more sense Right, so that's got, I'll hand it over to Matt to kind of give his perspective on this. And we can use some of the stuff that we're doing with one of our clients right now, just in
22:58 concept, to kind of drive it home on the water solution that we're, sorry, water management solution. We're putting together for produce water management. But I'll turn it over to Matt. Yeah,
23:07 thanks, John. So how we interact with it, John's absolutely right in the fact that manipulating and interrogating the graph itself, isn't what majority of the users will see. It's the back end
23:20 that's behind there. The web interface or the local machine interface is fully customizable for the application that we're working through, right? So if we're building an advisor that's working
23:34 through a water management scenario, we still need to display all of the relevant data that that operations engineer, the drilling engineer, whomever is interfacing with that data set needs to see,
23:46 we've done some really cool things contextually that is a byproduct of us organizing the data, even though it's disparaged in the back end, we can map to it and bring all of that to the forefront
23:59 interface. So when it's sitting apparent, filterable, just like a normal data set would be, it's interesting, as an engineer, face value, it's interesting. We can then step into those data
24:11 sets and use AI interrogate those individual data sets and create summaries around the data that becomes really interesting. If I'm simply preparing for a workover for that day, I can key that one
24:25 well up and I get the full history of that well in natural language. And instead of sitting and trying to decipher tables and rows and how my data is related to each other, I get a context that's in
24:38 natural language back to us. Secondarily because of that organization and that underlining feature of the graph organization, we can use the language models to be able to interrogate it in your
24:52 similar chat GPT scenario and ask it really intelligent questions on how do we want to structure our day? How do we want to organize a plug-in bannerment for a specific well? We've got a multitude
25:08 of data sets that we can simply query and use it as a thought partner. to help create value for that proposition. Yeah, right, one last thing I'd just add to that is it's super easy from a user
25:25 interface perspective to speak in your own words, your own language, the way that you query a system sort of very naturally fits within how you talk about work on a day-to-day basis. And one of the
25:37 things that's really unique about the system that we built is it doesn't rely on the large language models to define sort of ontologies or terminology or other things. So we can see that knowledge
25:51 about how we're talking about something and what it means within context to those models. They can still use their training corpus to talk about how industry has potentially solved problems in these
26:04 spaces before, Because of us paving this road in a digital way for these models to see this environment in almost 3D, they don't hallucinate. And so we've essentially created a system where these
26:19 models are grounded by the sort of hyper-contextual reality that we provide it, and it's a byproduct of that, it doesn't have to go think about a ton of things. It can just leverage what's
26:30 appropriate to the question that we've asked and the information that we've provided it And so that's a little bit of a differentiation point for us is, you know, we still use large language
26:40 knowledge. It doesn't matter which one we use, because they're really, really useful for people to convey their thoughts and words about a thing that they're trying to solve. And that's probably
26:52 another point of differentiation for us. We're trying to make it super, super easy for people to ask really smart questions and then use the underlying context within the model. to define sort of
27:03 the inference associated to that, the constituent parts that make up what we need to leverage for drafting any sort of answers. And I think we've designed something that's gonna be very easy to use,
27:15 hyper-deployable, and at the same time, like really, really scalable. So, thanks, this last thing I'll say on that.
27:24 I mean, I have so many thoughts going through my head. First of all, I only know like 22 of what you're talking about, but I feel like I have a pretty good grasp of both oil and gas as well as
27:36 technology. And I think what you're talking about is a little bit more, more futuristic. When you guys go in and talk to like investors, for example, like how do you pitch this in a way that they
27:46 understand it? Right, because this is complex stuff that you're talking about. Like wait, I get it when you're talking to a technologist or somebody that's an oil and gas subject matter expert,
27:56 you get right into it. How do you pitch something like this to investors to say,
28:01 We don't have the hallucinations within our application. We can touch all these various data points and have you interact with it in a variety of different manners. I'm just sort of curious, like,
28:11 how do you do that to somebody that's not as familiar with oil and gas, with defense or with tech? Okay, I'll give you like
28:20 a two minute version of this that is as simple as I can make it. But with a caveat of saying, there is no investor or customer conversation that we've had, where we've sort of resolved what this
28:35 means in reality without showing people what it means and then talking about it at depth. And so, it's complex and quite frankly, it is sort of on the cutting edge of what people are doing in this
28:47 space right now. And so it's really paving new ground for lack of a better way of saying it. But in general, it's kind of a problem space, right? The problem space is that high reliability
28:58 industries like engineering and defense. or they're heavily regulated. They require decision making on bifurcated differentiated information and the water management things like a perfect example
29:13 because that requires wells information, production ops information, contractor information. We need all of the producing assets. We need to understand the contracts, the permits, everything
29:24 associated with that ecosystem, that network before we can define how we can interact with that network And that's really representative of high reliability industry in general. There's not usually
29:36 a sort of one clear line of sight thing that you're going to execute on without having to talk to other people in different functional groups. And so we really wanted to build a system that was super
29:46 flexible and integrating a bunch of different components, a bunch of different data types, and a bunch of different user perspectives so that we could have a well-rounded understanding of any given
29:56 problem space or any given network.
29:60 The other big problem in high reliability industry is like you generally tend as a byproduct of operating these environments, have exceptionally high thresholds for performance. You know, like
30:13 we're engineering a bridge. We're gonna need to know that that thing's gonna stand just a time before we let a bunch of people drive on it. You know, if we're engineering a new well in the Gulf of
30:24 Mexico, Deepwater Gulf of Mexico, we surely wanna make sure that we've engineered that in a way that is safe and has accounted for a number of contingencies. And defense is the same, you know, if
30:34 we're gonna execute operations in a foreign nation, if we're gonna do things that, you know, potentially have fairly serious and broad implications, if we wanna know for a fact that we're making
30:45 the right decision with the best possible information, with the best possible perspective that we can get at any point in time. And that's where these systems start to fail and that's where we start
30:56 to pick the ball up and run and our platform. At least as we understand it right now, is the only platform that offers fully explainable, traceable, and trusted interface. So you can see
31:09 everything. You can see the transactional density. You can see how information is connected, the value of those connections. You can see how information is leveraged whenever you ask a question.
31:19 You can see the intensity with which that information was leveraged to generate the answers that are being provided to people. Now this is like a full departure. Like if you ask Chad GPT today,
31:29 like how did you come up with your answer? It may give you some statistical reference for how a word was used in reference to another word. We're doing this across
31:41 like terabytes of information simultaneously. And so this is a full sort of step change and difference in approach. But our sales pitch really is like we built this solution. There is high trust.
31:55 high traceability, high transparency, and ultimately full cycle explainability. And that is an imperative for scaled deployment of generative AI solutions in an engineering environment and in a
32:10 defense environment. And so that's really the sales pitch. It's like, we're different, but we're different for that reason specifically. We wanna build things that work in those networks and to
32:22 make things that are gonna work in those networks, we have to have a reliability system. And that's ultimately kind of the sales pitch that we try to give people. Now, they're gonna say the same
32:34 thing that you're probably gonna say, which is, that's great, but I need to know a little bit more. And that happens every time. And that's the value of the conversation, right? And that's why
32:43 then you actually pull something up and show them a real life example, which you're not gonna do on a podcast. But Matt, I wanna flip it to you a little bit with you being the business development
32:52 sales guy. When you talk to oil and gas companies, we'll just focus on energy. When you go in and talk to oil and gas companies, are the conversations centered around generically like how do we
33:04 apply AI to our organization or is it more specific? We have this one use case that is super manual. How do you take this
33:14 and have your robots do the work or what are you seeing a little bit more of the specifics or like the high level, how do we do this stuff? I really think the high level is probably what the
33:24 conversation boils down to. It's I have a problem or I have a tremendous amount of effort in a specific space in my organization that I've got an inclination. I wanna see if AI can help reduce that
33:41 load. I wanna see if we can enhance generational knowledge across my business. So how do we take Fred that's been the drilling engineer for the last 35 years And how do we compress all this?
33:54 information that he's acquired, all of his experience, and make that usable to interrogate on a real-time basis for the next drilling engineer that's walking in the door. So I think a lot of time
34:08 starts off with how are you doing it? But ultimately the real value in the conversation comes out to why are you doing it? And if we can come to terms with trying to solve that why, chances are
34:22 it's a win-win in both scenarios Very quickly. Yeah, I dig that. I think that's a question people should be asking more of because then if you don't ask that, you just continue to do business the
34:34 same way. Which as we all know, there's going to be better or cost effective technological advances that allow you to be more efficient as an organization. I'm curious a little bit, you know, one
34:48 of my good friends, CIO at a, a large publicly traded oil and gas company. And what he told me is, we're looking at right now with AI, kind of like what the internet was in the early 90s. Like,
35:03 we don't think this is a fad, right? This isn't going away. This is only gonna continue to get bigger and something that you have to use. And if you don't, you're going to be left behind, which
35:14 leads me to ask you guys, since you live in this world, like what does AI start to look like in three years, five years, 10 years. Like you're just getting into the basics of what it is, but
35:25 what does it look like when this starts to become like more mature or more evolved? Yeah, I think in a general sense, it's the promise of it is essentially a rising tide. And it's a rising tide
35:41 lifts all boats. We essentially elevate the starting point. The example that Matt gave is a common one, right?
35:48 knowledge management programs at work and oil and gas for a long time. Like Jeff, our CTO, and I even ran a program at work where we went around and interviewed all the experts and all the business
35:58 units that we worked in about drilling and completions, design work, and trying to understand their thought, process and application and contingency planning. You know, people have been trying to
36:08 do this for a long time. What these systems really hold the promise of is institutionalizing an approach to that but in an automated way. 'Cause you can leverage your information in such a hyper
36:21 tangible way that it really starts to be, okay, now that I know I have this information, like what can I apply it to? What sort of user perspectives can I look at this through? As a byproduct of
36:33 approaching it that way, you can essentially, if you do it right, in three years, take every new engineer that walks through the door and say like I, know you don't know much about what you're
36:44 about to get into, but. It doesn't matter because we built systems around you that are gonna support your ability to make great decisions right away. And it's sort of our philosophy is really
36:57 geared towards reinforcement learning in general. Like we've designed our platform to get better every day and to get better through engagement. And really that's a function of automation, that's a
37:09 function of algorithm deployments and really it's a function of understanding how subject matter expertise is applied and give them problem space. Think of it in a couple of years. We've got to have
37:22 an iPhone application.
37:25 The drill site manager, company man on the rig sitting there, stuck pipe happens like that. He can just query a natural language, stuck pipe event. I'm in this location. I need to know the
37:36 history of stuck pipe events like that. How did we resolve them? And within three minutes, get a detailed plan for exactly how these problems are manifested in the past, what we did to resolve
37:48 those problems, order first order actions they should take immediately and then produce an actual plan of action of how to remediate. You know, like that's a reality in a couple of years where
37:59 somebody can just go bang, bang, bang, okay, I need to solve this problem right now. And if we've mapped the relevant history associated to that problem space or the areas that they've worked in
38:09 and we've done it right, like now you have got essentially, I used to be a roving drill site manager in California. So I would go around whenever Riggs had problems. And I would just use my
38:21 knowledge because I was a pretty bad company man and a pretty bad engineer. I ran into lots of problems. I got lots of practice. And they were like, you've had so much practice with problems.
38:30 Like we're gonna send you out to solve problems now. That was just Rolodex, right? That's just like mental heuristics. Like I understand this problem. I understand how to solve this problem.
38:39 I've seen it enough at this point. You know, we hire 30, 40 year guys to sit around to do exactly that today. And if we can institutionalize the way that they think the way that they problem solve,
38:52 and their historical knowledge, and it doesn't even have to be theirs. It's like the knowledge of the area. Well, now that's a rising tide that lifts all boats Like we can then, at that point,
39:04 say, hey, as an organization, we have hyper-predictability and how we address problems. And I think that we're a few years away from that. I think that what we're doing at DataSquares is on the
39:14 leading edge of pushing us in that direction. I don't think the general applications for like chat, GPT, and some of these other things are gonna get us there anytime soon. I could be wrong. So
39:26 don't take that as gospel. But at the end of the day, That's really the promise that it holds and the potential that you could see in fairly short order, three to five years, if people are really
39:39 cognizant about the way that they approach this, they're going to win. They're going to rise all of their people up to a level and it doesn't matter if they're day one in the system or not. One of
39:54 our professors at HPS has this great quote, it's like, AI is not going to replace humans, but humans with AI are going to replace humans without it. So I think your CIO friend is right. Like, I
40:09 think he's on the right track. I don't think this is going anywhere. But what that means is the people that spend the time to try to figure this out and leverage it right now are going to be really
40:19 far ahead of the people that don't. Because there's nothing but promise and potential that's building every day that we pass There's new releases of technology tools and things that you can leverage.
40:31 just a new arrow, you add to the quiver. And this is sort of the imperative. Like if you as an organization want to compete on margins, like you really need to start looking and thinking about how
40:43 you can leverage some of this stuff. And the fun thing is there are lots of places that you can leverage it, tons. The conversations that we've had at this point, really strategic in nature, but
40:54 there's real tactical application. Like that's not five examples that I gave you Some of the other stuff that we've talked about, like how do I acquire the right leases within the structure of what
41:04 my portfolio strategy is? How can I avoid lost production? What are my opportunities in that space? What does a lease operations expense advisor look like? How can I look at sort of the network of
41:19 operated assets and understand what that costs me and how to lever those assets to increase revenue and reduce costs? Well, abandonment advisory, Well Engineering Advisory, Well Completions
41:29 Advisory. I mean, if I could go into an area, this is the fun part, it's not deterministic, right? And it's not, hey, what have we done and why will that work? It's like, what have we done?
41:39 What are the current conditions? And using all of that institutional knowledge, designed me a new way to maximize my potential in this area. Like, that's the real lever. It's not, tell me what
41:52 we've done before and what works best. This is, tell me what we've done before, what works best, take current conditions and design something new that's gonna work better. Like, that's the
42:02 promise. Like, that's where you start to get into something that's really needle moving and how we as an industry sort of approach problem solving in general, but it's sort of a full cycle thing
42:13 that has the ability to grow in a lot of different ways that are really positive. But the people that take the time to try to understand it, test it, find those areas of opportunity now are gonna
42:25 be the folks that are gonna
42:28 win. Matt, you want to chime in? I think just what I want to close that with is it's going to and it will create an ability for us to solve complex problems in a more efficient manner. I think
42:44 that holistically boils down what we're trying to do. We can throw manpower at complicated problems. We can throw late nights and lots of hours at complicated problems and really talented people,
42:56 how do we simplify that, increase the quality of performance that comes out of it, and increase the speed at which we can solve complex problems. The multitude of problems John just laid out there
43:10 that takes years to gain the knowledge, months to gain the data, and the confidence to be able to solve those problems If we can boil that down into the ability for the generic engineer that's super
43:25 skilled makes great decisions, to have another tool in his toolbox to make him faster and better, we're all gonna win out of that. No doubt. So just one more kind of AI question that I wanna shift
43:37 a little bit here is,
43:40 so you're a big company, right? You're a big operator and you wanna start applying AI. You pick a specific use case, you go with that, you have the budget to do it. You can talk about it and
43:50 tout it in your 10K and then your investor calls make sense What would you say to the really small operator, right? Should they be applying AI? Should they be looking into these things? Is it more
44:02 critical for them? And like, how do you convince the little guy that you actually should be doing this and this is your competitive advantage over the big guy? I've got, Matt and I are gonna have
44:14 different perspectives on this because I worked at big, big companies, right? BP and Chevron for the entirety of my oil and gas career I
44:24 think companies have a certain. amount of inertia that you have to overcome to do anything really interesting. And, you know, as a byproduct of that and how differentiated they are and how like
44:38 really geographically dispersed they are, you have pockets of approaches and you know, there are gonna be people that try to tackle things. Where I think this stuff makes
44:49 the biggest impact is not in those companies. It's not saying they can't benefit from it but the wide-scale sort of applicability across the board is like, it's an old capability multiplier. How do
44:60 we do more with the people we have without adding head-down or GNA in general? And what does that mean for my ability to be more predictable? And in general, it boils down to a couple of principles.
45:10 How can I reduce cost and increase revenue? That's really it at the end of the day and companies that operate on the margins. Those are gonna be the companies that win the most 'cause they're
45:21 already in a mindset of, I need to do more with less. And as a byproduct of that, they're always seeking ways and opportunities to optimize how they work, who they work with, what they do, and
45:34 how efficient they can be. And that's like the perfect mindset to start with when you approach these things. There are a lot of like low hanging fruit things, but I think in general, if you just
45:44 approach it from, where are my opportunities to scale my business up without adding head count and reduce complexity and increase predictability? Like if you just sort of look at it in those
45:56 measures, like mid-tier EMPs and small operators are always operating on the margins. And the more we can do to increase productivity, increase predictability from that productivity and reduce
46:09 volatility, those people win more. And that's sort of the perfect lens that I think I see this world through. Not that Chevron or Exxon or Shell won't benefit from some of these things, but you
46:22 almost have to look at them a business unit by business unit approach, 'cause the operations are really differentiated, the ways that they operate, the JOA's, the PSC's, all the things dictate
46:31 sort of differentiation. So they're their own businesses in general. So you have to find a business partner that you'd wanna tackle in a business unit that makes sense. Smaller companies are
46:42 already that business unit. They're already focused on the margins. I think that's really, in my opinion, where I see the biggest bang for the buck And so I think folks are gonna win a lot if they
46:56 sort of focus on that and at that level. Again, like I'm not saying I don't wanna work with Chevron Exxon or Shell at the end of the day. If anybody's out there listening, we're happy to talk.
47:05 I'm sure you're in the middle of it.
47:07 Yeah, exactly. But I do think this is the real force multiplier, the real or capability multiplier for mid-tier, small companies that are trying to grow and trying to do that in a fiscally sound
47:21 and predictable and repeatable way.
47:24 Now, I couldn't agree more, John, and I think the perspective of the large corporation of Chevron, in my case, my applicability is trying to move GE, my time with GE there, trying to adopt
47:39 something like this. It has to, just like you said, be boiled down into a specific business unit and then turn or nurture a use case at that point This mid-tier or smaller operator that has the
47:53 capability to be fast and be flexible but at the same time is resource constrained in a lot of different avenues. We're all wearing so many different hats, whether that's, I'm trying to manage a
48:05 regulatory issue on one side with a multitude of PAs that I need to manage and that doesn't make me money so it's on the side of my desk even though it will get done. It's extra hours to resolve that
48:19 If I can free that space up as CEO or as a COO of a smaller tier operator. I've got so much more tools in my tool belt to solve problems faster if I adopt AI sooner. I'll give you a good example and
48:38 this probably I'll give to and I'll try to be exceptionally brief and how I talk about this. But just from an org capability multiplier, go to a government use case. So we were lucky enough to get
48:50 some CIA analysts like test data and we were participating in a government catalyst accelerator program out of Colorado Springs. We needed a government use case because we had really only focused on
49:03 oil and gas at that point. So we got this thing which is a capstone program for analysts that are graduating their training and development programs and then going into their full time jobs and we
49:13 have to sort of do this before they can progress to that. Usually takes a team of six analysts, six weeks to do. this case work, and it's differentiated information, it's geolocation information
49:28 that's completely differentiated and bifurcated from human intelligence reporting, which is words, you know, people writing down signals, intelligence, and then financial transactions,
49:37 intelligence. And so you have phone records, banking transactions, locations, people's names, human reports, and a bunch of different stuff, and it is really hard to work through. So six weeks,
49:47 six people, average score on this is about 70 You get 70 you're doing good. We took that information as a test case, and I remember it was just so funny. This is actually a big moment where we go,
49:59 Oh, we're on to something. So we took this, we were working with a professor who was a friend and advisor of ours. This is all fictitious data, so I'm not saying anything, but there's a couple
50:12 of real main things that you try to solve. It was like, who killed the confidential informant, who paid for it, and what attacks are they planning next? Great, so CIA, Interesting International.
50:23 stop. So we did that. We took the data. We took it back to him the next day and said, hey, we're going to review what we've internalized. We've set it up. We just want to show you what we have
50:33 that way. We know we're like, on the right track, we got it all in the system correctly. I remember him saying like, can't be right. And that's going, yeah, we know we did it 14 hours ago. We
50:45 know it can't be right. And he goes, no, no, it can't be right because I'm asking it questions in natural language and it's not gotten anything wrong yet. And he goes, I want to spend a little
50:55 bit more time with it. So a couple hours later, he called us back and he goes like, I don't know what you guys did, but you solved this in less than a day at better than 100 because your system
51:06 identified 59 connective issues in the data that I didn't know existed and I wrote it And so we essentially solved it at about 120 because we added about 20 granularity and actually sort of fidelity
51:23 in the data model in less than a day. And so just keep that in your mind. So six people, six weeks average score, 70, 14 hours, better than 100, solving really complex data differentiated
51:38 problems. So that's sort of one org capability multiplier example. Another one I'll give you is like a hard question that we were asked by one of our customers right now And it said, I want to
51:51 optimize my disposal activities. I want to maximize on profitability for my SWD assets. I want to make sure that I understand what my high disposal assets are right now, what my low expense per
52:02 barrel assets are, where I have skim potential. This is not an easy question to answer. There's like four other factors. I want to answer that question. I was like, oh, that question is very
52:13 hard to answer because it's really difficult to put all these pieces together. Sure So we can ask the system today. That same question, and within 10 seconds, we will get an answer that says like,
52:26 here are your assets, here's your current yields, what your expense per barrel is, where your skim oil potential is, here's the revenue that can be generated from that skim oil deployment, here's
52:36 your cost, here's your cost-oiled or profit-oiled breakdowns on that deployment of capital, here's your high permit volume utilization wells, their current utilization, their price per barrel on
52:47 the supposed, like, generated income, and then low-expense operated assets that fit within the structure, and it's sort of combining all of these things and saying, Okay, here's your answer.
52:58 Like, my recommendation is you pay attention to these five wells for deployment of this, you reduce your ops costs by taking some of your activities off of the assets that cost a lot to run, you
53:08 maximize your skim oil potential by deployment into these three assets, which should generate about 29 million in revenue next year as a by-product of this decision, and you utilize your capacity on
53:19 this, which is gonna create another 30 million in total revenue as a byproduct to that over the course of the next year. It's like being able to answer that question, which is very difficult, very
53:32 quickly, is an example of force multiplication. It's essentially taking 10 different units of information and 10 perspectives on operated assets and production and all these different things and
53:44 going very quickly, sort through it all, use the network, your understanding of it and all the connections and what they mean monetarily for me, the operator, to tell me how to operate these
53:56 assets more effectively. That's right. What we're doing now is trying to automate that. And we're really close to being able to automate that so that you can actually just deploy this and say like,
54:08 Hey, I wanna optimize on this lever today, all right? Toggle it, now let's go out here deploy that as the new plan of activity for the next, you know, two weeks.
54:19 Shish. Okay. I got two more questions. Be relatively brief. Yeah. What, what would you, what advice would you give to like the early career professional today, right? Somebody that wants to
54:31 get into oil and gas or somebody that wants to get into technology and has an inkling around wanting to leverage AI. What would, what would you tell that person to focus on? Yeah, we'll have
54:43 different perspectives on this
54:46 I think. Might be, might be really quick. I didn't come to sort of the engineering aspects of this, like very naturally, like I didn't really want to study engineering. I saw that as an
54:58 opportunity, as a byproduct of, you know, you talk to some friends, hey, you're working in oil and gas, how much money do you make? Oh, maybe I should do that kind of thing. But for me,
55:12 you have to approach, I think, your role in this space as a learning opportunity on a day to day basis. Like every single day, I try to understand something new about the industry, something new
55:24 about the way that it works, the connections and how technology is being used. And really, for me, like the last decade of my career was an everyday learning opportunity because it was like, go
55:34 problem solve things that are really differentiated in nature. And then given sort of a remit to try to figure out how to do that and persist some of those solutions through technology, which again,
55:46 opens up a door of like, I don't know enough about this and I need to learn more about it. So that's kind of part one, be a self starter, be a self learner, continue to approach learning as an
55:55 everyday task and thing that you need to do just like working out needing healthy. The other part of that equation for me is seeking out expertise, right? And I just don't like as a young person
56:07 coming into a company, approach everything through the lens of who are the experts? What do they know and how can I learn from them? And for me, like that was my whole approach to everything in my
56:19 career. It's like continue to learn every day and learn from people that know best. And if you can do that through the lens of technology, like you're gonna be set up 'cause this digital engineer
56:32 thing that started coming up about a decade ago, that's not going away. So like engineers are gonna need to know how to operate in coded environments because that is the environment of the future So
56:44 like it's not all math, it's not all statistics. Like you need to know some of those things to be fluent in these spaces, but at the end of the day, just continue to be an engineer and approach
56:54 your day-to-day life. Like I need to learn something new and I need to learn it from the right people. And if you do that, you're gonna be successful. Love it. Yeah, I'll follow that up. I
57:02 think we're pretty similar there, John. A couple of things that, and I look at through the lens of how am I advising? I've got three teenage daughters here at the house One's a senior getting
57:14 ready to head off to college. And so how are we thinking about where to go? And if we take that four or five years down the road, what advice are we giving them? And I think it's the ability to
57:27 embrace technology, but still have some level of skepticism and ground in reality, right? We have to trust the knowledge that we have as individuals. We have to go build that skill set So we have
57:42 a really good corpus of knowledge to know what's right and what's wrong as we start to apply fast solutions. The AI is not going to be to the point where in the next three to five years, we can
57:55 completely trust what's coming out of it as a mass product. And we're gonna see it in every part of our life. But the ability to understand how to use it as a tool to make yourself more effective
58:09 and more employable I think that's. that's paramount for us to be able to be successful going forward. Nice. Final question, where can people find you guys, whether it's LinkedIn, websites,
58:22 social, all those sorts of things, if people want to learn more about you too and your company? Yeah, the website is data2ai and
58:33 our handle or whatever
58:39 for
58:40 LinkedIn is at Data2US So those are the two places you can find us. We don't really have a social media presence outside of that. We sort of use those as our avenues to engage with folks, but that
58:51 LinkedIn we're persistently available on and we
58:54 can be contacted through and you can obviously contact us through our website as well. Yeah, apparently you can get jobs through LinkedIn too. There you go, look for Matt. I appreciate you guys
59:05 coming on today. I think this will be a pretty widely listened Two episodes since the topic is one of extreme curiosity. I learned a lot, I'm going to have to rack my brain after this to think
59:16 about all the things that I did learn, but ultimately, I think what you're doing is in some ways revolutionary in more ways, it's just logical, it's leveraging tools that exist to make businesses
59:28 more efficient and better, and not replacing people, but making people better
59:34 at their job. So keep doing what you do, data squared team, appreciate you guys, thanks for coming off
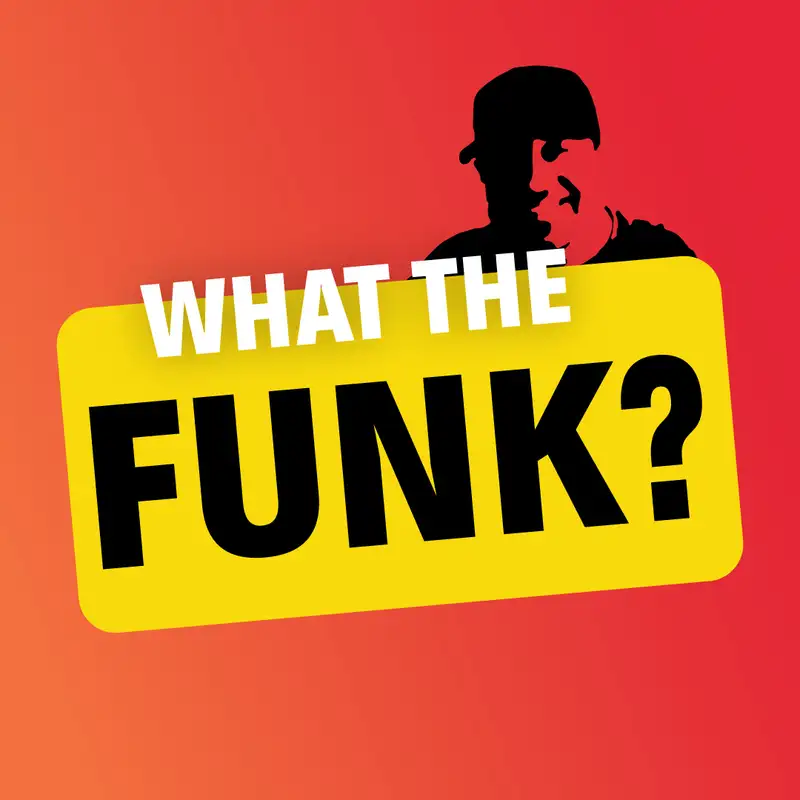